A Guide To Understanding Contrastive Pre-Training: Revolutionizing AI Training Efficiency
A Guide To Understanding Contrastive Pre-Training: Revolutionizing AI Training Efficiency
Introduction
The realm of artificial intelligence continues to evolve at an astonishing rate. As we strive for more advanced AI models, innovative training methodologies have become essential to enhancing performance and efficiency. One such revolutionary approach is contrastive pre-training. This guide will walk you through the principles of contrastive pre-training, its applications, and the immense benefits it can yield for organizations seeking to enhance their AI training processes.
What is Contrastive Pre-Training?
At its core, contrastive pre-training is a transformative method in deep learning that improves the utility of labeled data. It focuses on unsupervised clustering of unlabeled images in the latent space, effectively defining decision boundaries between distinct classes. This means that instead of relying solely on extensive labeled datasets, contrastive pre-training allows models to learn from unlabeled data, thus enhancing the overall efficiency of the training process.
Applications in Visual-Language Models
Visual-language models like CLIP (Contrastive Language–Image Pre-training) are excellent illustrations of how contrastive pre-training can bridge textual and visual information. Utilizing a dual-encoder architecture, these models efficiently map images and text into a shared latent space. During training, the model processes pairs of images and text captions, distinguishing between genuine matches and mismatched sets. The contrastive loss function plays a critical role here, encouraging the model to prioritize correct associations while penalizing inaccuracies.
Benefits of Contrastive Pre-Training
- Improved Efficiency with Labeled Data: By leveraging unsupervised clustering, contrastive pre-training lessens the dependency on extensive labeled datasets, resulting in a more efficient training process.
- Enhanced Performance in Zero-Shot Learning: Models like CLIP showcase remarkable capabilities in zero-shot learning, allowing accurate predictions even without explicit fine-tuning for new tasks, thus facilitating quicker adaptability.
- Robustness to Image Corruptions: The resilience provided by contrastive learning methods ensures robust performance across various scenarios, making these models reliable in diverse real-world applications.
- Applications Beyond Computer Vision: The impact of contrastive learning extends into natural language processing (NLP) as well, improving sentence embeddings and language understanding efficiency.
The Role of Employee Training in AI Adoption
While contrastive pre-training undoubtedly enhances AI models, its true potential is only realized when paired with a well-informed workforce. Employee training is paramount for several reasons:
- Understanding AI Technologies: Employees must grasp the methodologies behind AI training, including concepts like contrastive pre-training and their applications across various domains.
- Data Preparation and Management: The success of AI training lies in effective data preparation. Employees trained in proper data management ensure that it is primed for models like those utilizing contrastive pre-training.
- Model Interpretation and Deployment: Once an AI model is trained, it’s crucial for employees to interpret results accurately, identify biases, and deploy models aligning with organizational goals.
- Continuous Learning and Adaptation: With AI’s rapid evolution, continual learning is essential. Organizations must foster an environment of ongoing education so employees can stay abreast of the latest methodologies and innovations.
Conclusion
Contrastive pre-training offers a considerable advancement in AI training methodologies. By employing unsupervised clustering and contrastive loss functions, organizations can capitalize on unlabeled data, reducing dependence on vast labeled datasets. The remarkable implications of this technique extend into several fields beyond just computer vision, impacting natural language processing and more.
Ultimately, the real power of contrastive pre-training lies in its synergy with a competent workforce. By investing in employee training, organizations can harness the advantages of this innovative approach and drive efficiency and creativity within their operations. Take the leap into AI training; equip your entire workforce with the skills needed to thrive in this transformative landscape. Explore comprehensive training programs and certification opportunities to elevate your organization’s capabilities.
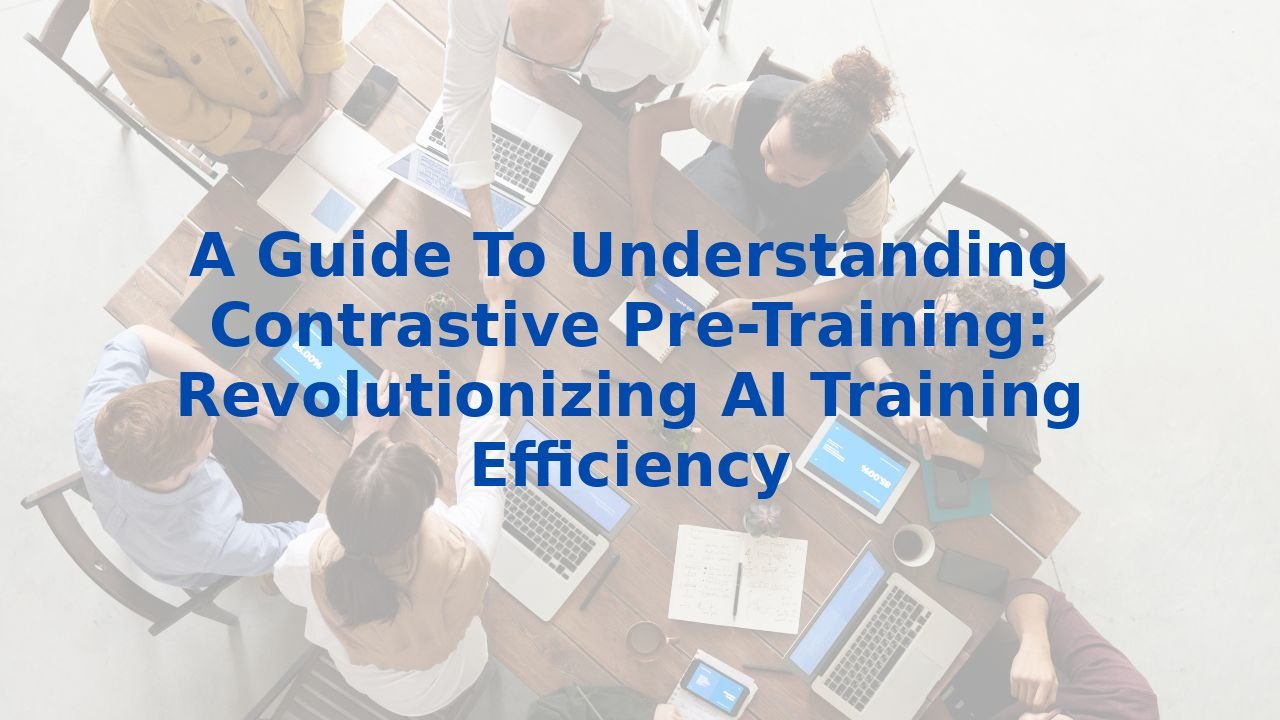