Everything You Need To Know About Navigating Data Science Taxonomy and Process Models with AI
Navigating the Taxonomy and Process Model in Data Science with AI
Data science and analytics are at the forefront of innovative business processes, and understanding the underlying structure is crucial for leveraging machine learning effectively. This blog post delves into the essence of data science taxonomies and process models, emphasizing how artificial intelligence (AI) can enhance these frameworks to improve organizational efficiency.
Understanding Data Science Taxonomy
A data science taxonomy systematically organizes and classifies the complex concepts and processes that define the field. Imagine a structured library where every genre and book is categorized for seamless access—that’s what a well-defined taxonomy does for data science. By enabling professionals to communicate ideas clearly, it fosters collaboration and inter-departmental synergy, a key aspect of modern businesses.
The Structure of a Data Science Process Model
The data science process model is comprised of several key phases: data acquisition, preprocessing, modeling, evaluation, and deployment. Each of these phases has its tools and methodologies tailored to ensure a smooth transition from raw data to meaningful insights.
"AI acts as the backbone of these phases, automating repetitive tasks and allowing analysts to focus on strategic decision-making."
For instance, AI enhances data acquisition by efficiently collecting vast datasets from multiple sources, reducing time and human error. During preprocessing, machine learning algorithms can identify and rectify data quality issues, ensuring that the information is clean and ready for analysis.
Machine Learning's Role in Data Analysis
Machine learning serves as a transformative pillar in the data science process. By analyzing historical data and patterns, machine learning models can make predictions that inform business strategies. These advanced algorithms can be tailored to various industries, enabling businesses to customize processes to meet unique market needs.
Furthermore, the integration of AI into data analytics means that businesses can evolve from descriptive analytics—what happened—to predictive analytics—what is likely to happen. This evolution not only enhances decision-making but also drives innovation, as insights derived from data empower teams to develop proactive strategies rather than reactive ones.
Common Challenges and Overcoming Them
The journey through data science is not without its challenges. Data quality issues, model interpretability, and the ever-evolving landscape of technologies can pose significant obstacles. However, these challenges can be systematically addressed.
Implementing robust validation processes helps in mitigating data quality issues, while investing in employee training on model interpretability can foster a culture of informed decision-making. This is where AI can really shine—by offering intelligent solutions that provide clarity amidst complexity.
The Efficiency Boost from AI
AI-driven automation of data tasks doesn’t merely expedite processes; it also enhances accuracy and consistency. For organizations, this translates into significant—sometimes game-changing—efficiencies. By freeing employees from mundane analytical tasks, AI empowers them to engage in higher-level, strategic planning initiatives that can drive growth and innovation.
Moreover, machine learning’s ability to continually learn and adapt allows businesses to stay ahead of competitors. Organizations that embrace AI are not just responding to market needs; they are anticipating them, laying the groundwork for proactive rather than reactive business strategies.
Investing in Employee Training for AI Integration
To maximize the benefits of AI implementations, organizations must consider the necessity of training their employees in AI technologies. Upskilling staff not only broadens the internal expertise but also fosters an adaptable workforce capable of navigating the complexities of data science and evolving technologies. Training equips employees with essential skills, paving the way for seamless integration of AI into existing processes and ensuring that teams can harness the full potential of machine learning insights.
"In investing in people, organizations cultivate resilience; adaptability ultimately becomes a competitive advantage in an increasingly digitized world.”
Conclusion: The Path Forward
As we navigate the intricate landscape of data science and analytics, understanding the taxonomy and process models is essential. By integrating AI into these frameworks, organizations can streamline processes, enhance decision-making, and ultimately surge ahead of the competition. Moreover, empowering employees through comprehensive training ensures that businesses are not only prepared for today’s challenges but also poised for tomorrow's opportunities.
For those looking to deepen their understanding of data science and AI, the evolving landscape beckons. The opportunity to engage in transformative learning experiences exists—will your organization seize it?
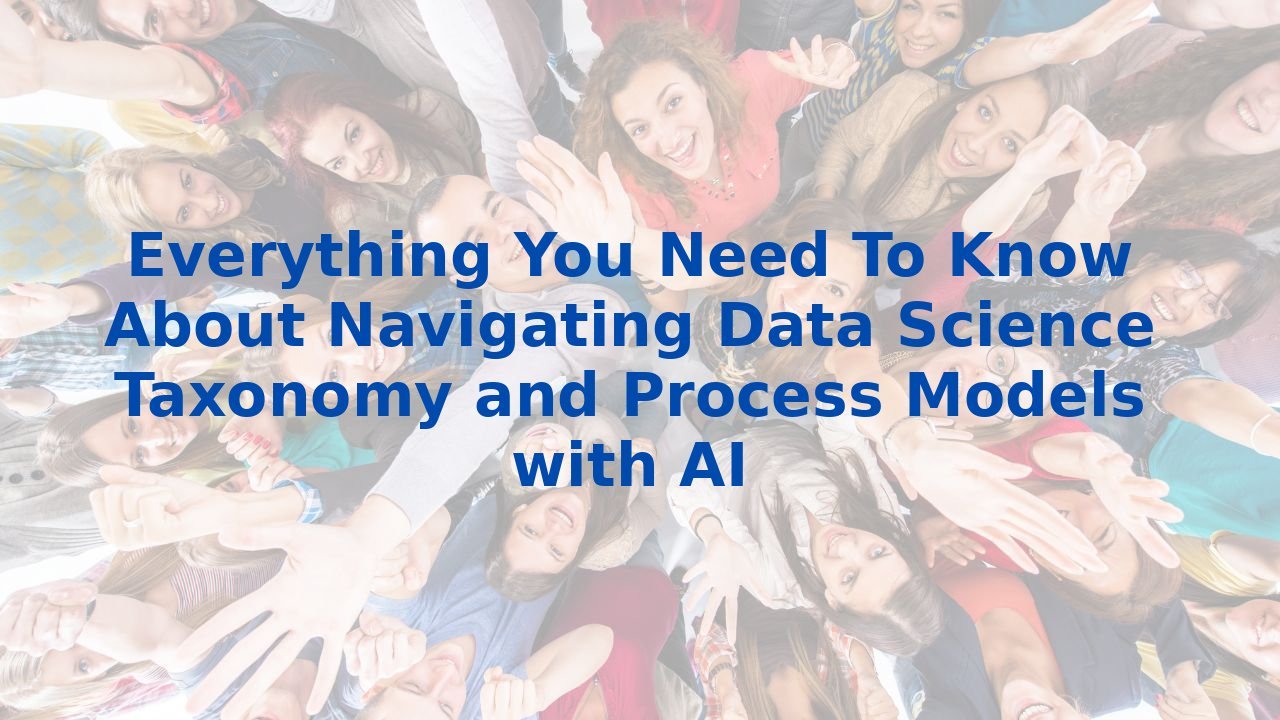