How To Optimize AI Model Performance: Unlocking Efficiency and Accuracy
How To Optimize AI Model Performance: Unlocking Efficiency and Accuracy
In today’s data-driven world, the promise of artificial intelligence isn’t just in its capabilities but in how well we can refine and optimize these capabilities to improve our business processes. Navigating through the complexities of AI optimization is not just a technical task; it’s a gateway to enhancing operational efficiency and accuracy across an organization. Let’s explore how you can optimize AI model performance and unlock its true potential.
The Importance of Optimizing AI Model Performance
At the core of any successful AI initiative lies the optimization of its models. This isn’t just a technicality; it’s a necessity that can lead to competitive advantages. Optimizing your AI systems is essential for improving operational efficiency, decreasing costs, and increasing the accuracy of insights generated. Through effective optimization, businesses stand to benefit in several profound ways.
Why Optimize AI Models?
One major reason to focus on AI optimization is to enhance operational efficiency. By reducing computational costs and improving resource allocation, businesses can ensure that their AI models run smoothly even under heavy data loads. Additionally, optimized models improve accuracy and reliability, ultimately leading to more informed and strategic decision-making. The real-world applicability of AI is directly tied to how well it can generalize, making optimization techniques critical for practical outcomes.
Key Techniques for AI Model Optimization
Understanding the fundamental techniques for optimization can empower organizations to make informed decisions. Here are some key strategies:
1. Hyperparameter Tuning
This involves tweaking parameters outside the learning algorithm itself, such as learning rate and dropout rates. Hyperparameter tuning can significantly affect how well your model learns, leading to better outcomes.
2. Data Preprocessing
The quality of data drives the quality of AI. Techniques such as normalization and feature scaling can mitigate noise and improve data quality. This foundational step lays the groundwork for effective learning.
3. Model Pruning
Eliminating unnecessary parameters simplifies the model, which can lead to lower resource consumption. This technique is especially crucial when deploying models on devices with limited capacity.
4. Retraining on Better Data
Outdated models lose relevance. Providing your models with high-quality, diverse, and current datasets ensures they continue to produce accurate outcomes, adapting to an ever-changing landscape.
5. Deployment Modification
Sometimes, a simple redeployment of a model on more capable hardware can bring immediate performance gains. However, addressing core optimization through model adjustments is crucial for long-term efficiency.
6. Source Code Enhancements
In some cases, modifying the underlying code of your model can yield substantial performance benefits. While this requires significant effort, retuning a model to new parameters can enhance its operational capabilities.
Benefits of AI Optimization for Business Processes
So why should any organization care about optimizing AI models? The benefits are abundant:
- Enhanced Efficiency: By streamlining resource utilization and cutting down on computational costs, optimized AI systems help organizations save money and avoid system bottlenecks.
- Improved Accuracy: A well-tuned model yields reliable predictions, facilitating better decision-making, especially in mission-critical operations like fraud detection or predictive analysis.
- Adaptability: An optimized model adapts more readily to new data and changing conditions, making it a dynamic asset for any organization aiming to stay ahead.
The Role of Employee Training in AI Optimization
While technological tools are essential, human involvement is equally critical. Training your employees in AI optimization techniques brings visible benefits:
- Understanding Optimization Techniques: Employees equipped with knowledge of optimization can ensure that AI models are designed for success from the beginning.
- Data Quality Management: Comprehensive training fosters a keen understanding of data relevance, thus enhancing data quality management and utilization.
- Model Maintenance: Regular maintenance of AI models requires a significant understanding of their underlying algorithms. Trained employees can perform these tasks seamlessly, ensuring sustained performance.
Conclusion
Optimizing AI model performance is not merely a technical necessity but a strategic advantage that drives organizational efficiency and accuracy. By utilizing advanced techniques like hyperparameter tuning, data preprocessing, and ongoing training of personnel, businesses can elevate their AI initiatives. When organizations commit to focusing on these optimization strategies, they unlock the full potential of their AI assets, propelling their processes to new heights of efficiency and effectiveness. In a world where data is king, optimizing AI is your pathway to reigning supreme.
For those looking to further enhance their capabilities, consider exploring comprehensive AI training solutions that equip your workforce with the necessary skills to master AI optimization and its many dimensions.
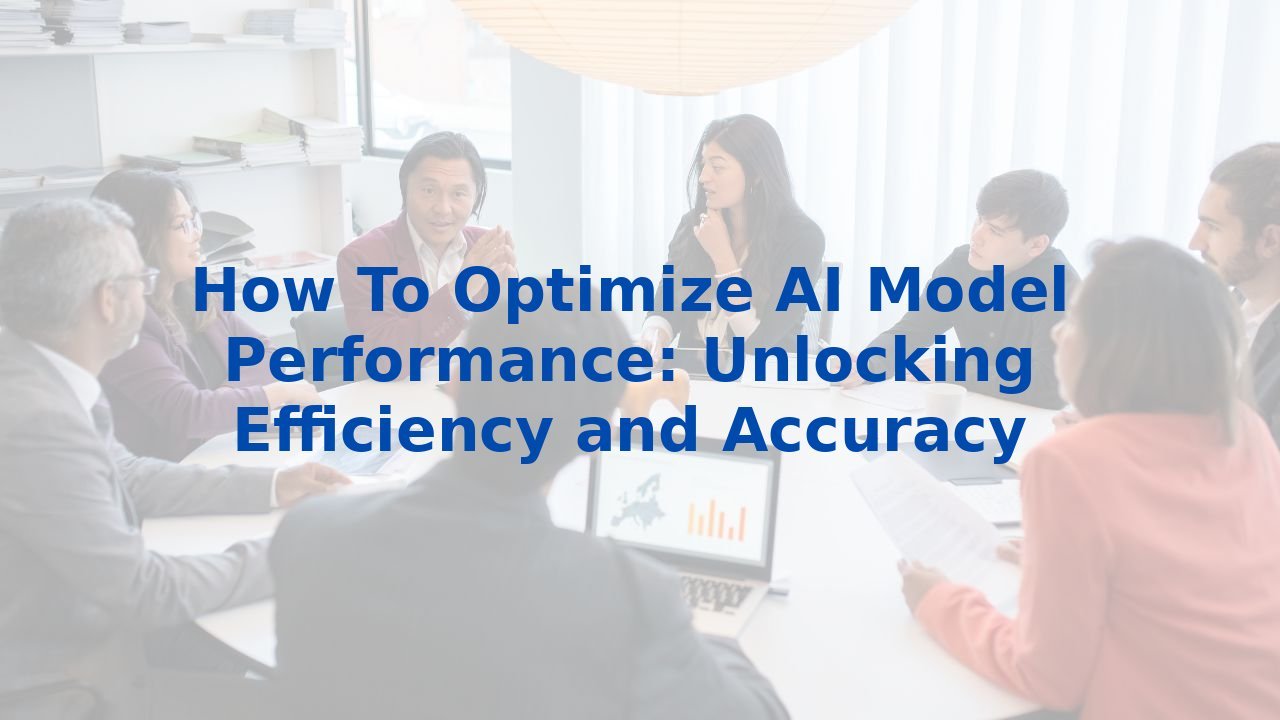