Navigating AI Bias: A Comprehensive Guide to Understanding and Rectifying Training Data Challenges
Navigating AI Bias: A Comprehensive Guide to Understanding and Rectifying Training Data Challenges
The Complexities of AI Training Data: Balancing Reality and Bias
Artificial intelligence (AI) is transforming industries by driving innovation, enhancing efficiencies, and allowing organizations to streamline their operations. Yet, as we propel forward into an AI-centered world, we're met with a challenge that cannot be overlooked: bias in training data. This guide will explore not just the implications of bias, but also how understanding and rectifying these challenges can enable businesses to thrive.
The Problem of Bias in AI
While AI holds incredible potential, it is not devoid of pitfalls. At the core of AI's challenges lies the issue of bias. Bias can stem from several sources, leading algorithms to produce results that don’t accurately represent the intended demographic or objective. The implications of this bias can be severe, affecting everything from hiring practices to customer engagement.
Sources of Bias in AI
Recognizing the sources of bias in AI is a step towards developing a more responsible approach in our organizations:
1. Data Bias: This is a primary culprit. If the training data lacks diversity, AI applications may yield skewed outcomes. An example can be found in facial recognition technology, which has been shown to struggle with accuracy when trained predominantly on data sets featuring white individuals.
2. Algorithmic Bias: Algorithms can perpetuate bias through factors like inconsistent labeling or the over-representation of certain attributes. For instance, recruiting AI could inadvertently sift out qualified candidates based on their irrelevant characteristics.
3. User Interaction Bias: AI systems learn from user interactions, meaning biases can propagate over time. For example, if users experience bias and incorporate it unconsciously into their decisions, this feedback loop reinforces the misconduct.
4. Cultural and Social Factors: Existing societal bias can seep into AI models, highlighting the need for a critical evaluation of how cultural perceptions shape data interpretation.
Debiasing Training Data
Correcting bias in training data requires meticulous attention and a thorough understanding of the interplay between data science and social understanding. Organizations must actively engage in reviewing their datasets, ensuring a diverse representation that mirrors reality rather than a singular narrative.
To successfully debias training data, businesses should:
- Audit datasets to identify and correct over-representations or under-representations.
- Employ data collection strategies that promote inclusivity.
- Work towards refining algorithms that can adapt to a broader spectrum of characteristics.
The Importance of Employee Training in AI
While rectifying biases in training data is critical, cultivating a workforce with a deep understanding of AI is equally essential. Employee training can help organizations harness AI's potential while avoiding its pitfalls.
Investing in employee training offers several benefits:
1. Understanding Bias: Educating employees about how biases arise in AI fosters a culture of awareness, enabling them to spot potential biases in AI systems more effectively.
2. Critical Thinking: Training nurtures critical thinking skills, allowing employees to assess AI outputs judiciously. This is especially vital in sectors like healthcare, where a misstep can have serious ramifications.
3. Ethical Considerations: Employees armed with an understanding of ethical implications can navigate the complex landscape of AI deployment, ensuring that tools are employed in ways that prioritize fairness and equity.
Conclusion: Striving for Equitable AI
The question of whether AI training data should reflect our imperfect reality or be adjusted to correct societal disparities is a nuanced discussion. However, the necessity of addressing bias in AI is crystal clear. By unpacking the sources of bias and actively engaging in the debiasing of training data, organizations can simultaneously improve efficiency and promote fairness.
As businesses strive to leverage AI as a transformative force, the commitment to train employees in AI's ethical and operational dimensions will be one of the most powerful strategies available. In fostering both technological advancement and social equity, we can shape a future where AI serves as a tool for inclusion rather than division.
To explore comprehensive programs facilitating such training initiatives, visit us at Complete AI Training.
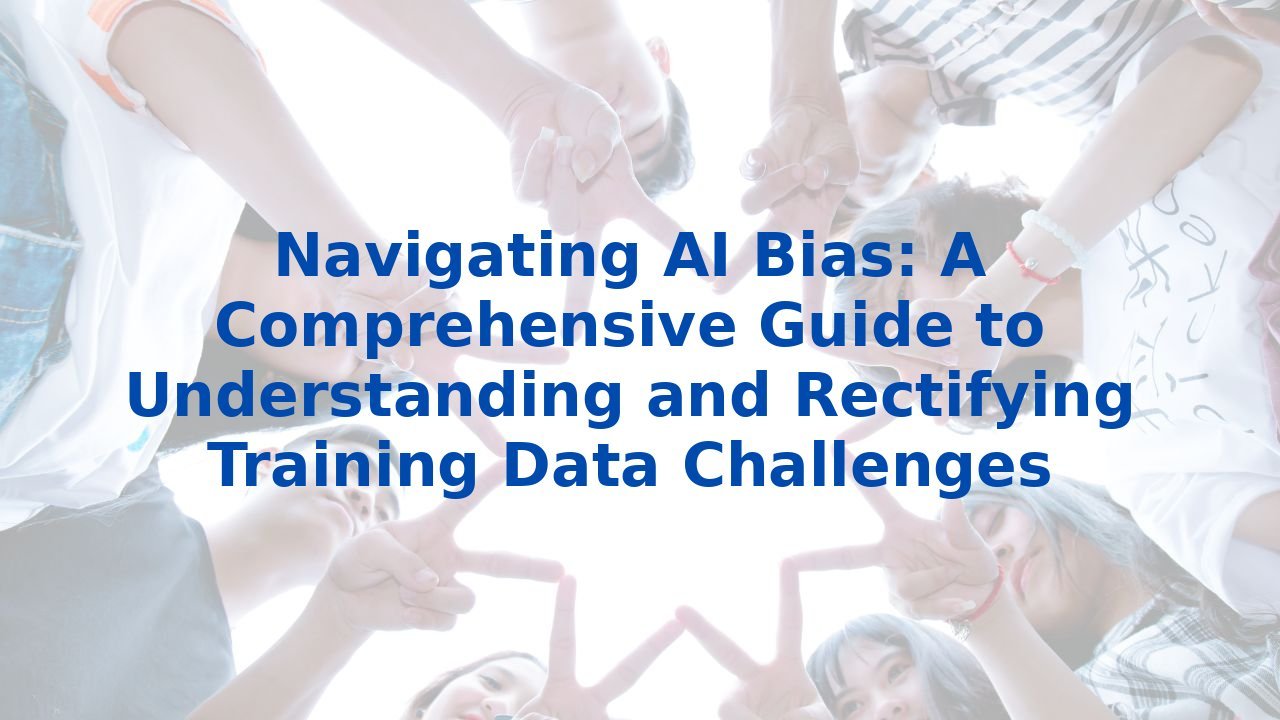